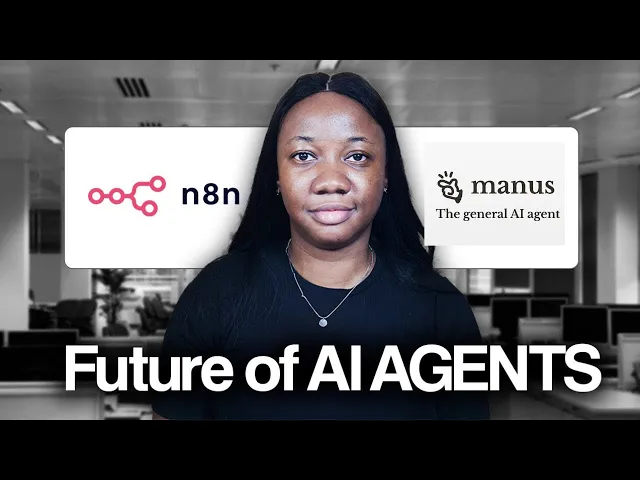
How AI Handles Dynamic Workflow Changes in Real Time
Apr 18, 2025
AI is reshaping how workflows adapt to sudden changes. Here's what you need to know:
Real-Time Adjustments: AI reallocates tasks instantly during spikes in activity, keeping operations smooth.
Better Resource Use: It balances workloads automatically to meet demand efficiently.
Consistent Quality: Service levels are maintained even during high-pressure situations.
Learning Over Time: AI improves by analyzing outcomes, ensuring workflows adapt to what works best.
NLP for Context: Natural Language Processing helps AI understand user intent, routing tasks more effectively.
Traditional systems struggle with rigid rules and manual updates, but AI-driven workflows thrive by processing live data, learning continuously, and integrating seamlessly with existing tools. This makes them more responsive and reliable in dynamic environments.
The Future of AI Workflow Automation | AI AGENTS
How AI Updates Workflows in Real Time
AI has transformed workflows by moving beyond static, rule-based systems. It constantly analyzes events and outcomes, adjusting processes on the fly. This lets it handle unexpected changes and sudden spikes in activity without requiring manual input.
Managing Live Data Streams
AI systems process event data as it happens, pulling information from various sources. They identify critical details - like call volume, wait times, or customer sentiment - and use that data to adjust workflows immediately. For instance, when a trend is detected, AI can create new workflow paths tailored to specific situations, ensuring resources are allocated effectively and tasks are routed efficiently.
Learning and Improving Over Time
AI doesn’t just follow a set of rules - it learns from its decisions. By collecting data on outcomes, such as resolution rates or customer satisfaction, it can refine its models. This feedback loop strengthens the system, allowing it to prioritize approaches that deliver better results. Over time, workflows adapt to what works best rather than sticking to pre-set guidelines.
Using NLP for Better Context
Natural Language Processing (NLP) helps AI understand user intent and the context of conversations. By analyzing how language is used, the system can route tasks based on factors like urgency, complexity, or the expertise needed. NLP also ensures smooth transitions between workflow stages by maintaining context, so conversations remain coherent even as tasks move through different parts of the process.
Next, we’ll explore how to prepare your data and integrate these AI capabilities into your current tools and systems.
Setting Up AI Workflow Systems
To create effective AI workflow systems, focus on processing live data and using NLP to capture context. Ensure your data and integrations are ready for real-time workflows.
Preparing Your Data
Start by reviewing support logs to gather a variety of high-quality historical interactions. Clean the data by removing any personally identifiable information (PII), standardizing formats, labeling outcomes, and conducting manual quality checks.
Pay attention to key details like customer intent, resolution paths, escalation triggers, and time-to-resolution metrics.
Integrating with Existing Tools
Identify how data flows between your CRM, ticketing system, and knowledge base. Set up real-time pipelines to handle interaction streams, update rules, sync context, and log decisions effectively.
Incorporate caching layers to reduce latency in these pipelines.
Testing and Improving
Simulate your production environment in a staging setup. Begin testing with synthetic data and gradually move to live interactions.
Monitor these critical metrics:
Response accuracy rates
Speed of decision-making
Error rates across different workflows
Automate feedback loops to identify edge cases, behavioral changes, shifts in resolution success, and performance inconsistencies. Set up alerts for threshold breaches and retrain your models if performance deviates from the baseline.
Common Problems and Solutions
Even with thorough testing, real-time AI workflows often encounter recurring challenges. Here's how to address them effectively.
Fixing Data Issues
Poor-quality data can throw AI workflows off track. Here's how to tackle it:
Real-time Data Validation: Automate checks for anomalies in data streams, standardize formats with cleansing pipelines, and set up fallback processes for corrupted inputs.
Data Synchronization: Maintain a single source of truth and use version control to keep workflow parameters consistent.
Handling High Volume
Managing high-volume demands requires smart strategies:
Use auto-scaling to handle spikes in demand and implement request queuing.
Build redundancy into the system to avoid downtime.
Monitor response times and error rates closely, and set up alerts to catch any signs of performance degradation.
Addressing Ethics in AI Automation
Ensuring ethical AI use is critical. Focus on these areas:
Bias Prevention: Regularly audit training data and decisions. Document and review strategies to minimize bias.
Privacy Protection: Encrypt data at every stage, enforce strict retention policies, and log all decisions for accountability.
Transparency: Clearly document decision-making logic and how edge cases are handled. Share this information with stakeholders to build trust.
Use unified dashboards to monitor both performance and ethical metrics, and review these regularly. Addressing these challenges head-on will help keep your AI system reliable and efficient, even under changing conditions.
Conclusion
Key Highlights
AI-driven workflows excel at handling live data streams, adapting to new information, and integrating with existing systems. These workflows offer benefits like automated decision-making, predictive adjustments, and the ability to maintain performance during unexpected changes or high demand. By continuously learning from outcomes, AI systems ensure reliable results even in challenging scenarios.
Fathom serves as a strong example of these capabilities in voice-based automation.
Fathom's Approach to Voice Automation

Fathom's infrastructure showcases how AI can handle dynamic, high-volume environments effectively. Their strategy focuses on three core areas:
Scalable architecture designed for high-volume, low-latency voice operations with dependable uptime.
Integrated routing combined with human-in-the-loop fallback to retain full call context when needed.
Voice-native observability through real-time transcripts, summaries, and resolution metrics.
As AI workflows continue to advance, they will become even better at anticipating changes, leading to greater resilience and efficiency.